Abstract
Background: Over the years, the South African government has emphasised improving the metros’ socioeconomic infrastructures because these form an essential catalyst that can boost grassroots development. Despite the considerable increase in investments in transport infrastructure in the metros, the contributions of the metros where all these transport investments are concentrated appear to be making little use of it to promote their grassroots development.
Objectives: The study investigated the impact of public transportation on the output growth of South Africa.
Method: One-way error component panel analysis is adopted to analyse disaggregated data from eight major metros in the country from 2003 to 2017. Data were mainly sourced on public transport expenditure, total social infrastructure expenditure, total capital formation, labour expenditure and output growth rate from each of the metros.
Results: The results were a clear departure from what was obtained by previous studies on transport expenditure and the growth of South Africa. Six of the metros which are the big ones in terms of the population showed a result, which indicated that public transport expenditure did not influence their output significantly; but when combined with other social infrastructure, it exhibited significant impact. However, the results of the remaining two small metros showed that public transport expenditure and its combination with other social infrastructure all had a significant impact on their output growth.
Conclusion: This confirms transport infrastructure investment conforms to the theory of the diminishing marginal product of capital. The six big metros should invest more in social infrastructure, which would complement the contemporary transport infrastructure investment. On the other hand, there is still a need to increase public transport infrastructure investments on the smaller metros.
Keywords: public transport expenditure; output growth; South African metros; public investments; social infrastructure.
Introduction
Over the years, the South African government has been emphasising on improving the socioeconomic infrastructures at the metros because these form an important catalyst that can boost grassroots development (Mayekiso 2015). Paramount amongst the social infrastructure is transportation because of its significance in the promotion of economic contributions of these metros to the overall economic growth of the country (Skorobogatova & Kuzmina-Merlino 2017). Social infrastructure investment used in the study, include government investment on health and educational institutions. Despite the huge increase in investments on transport infrastructure in the metros, the contributions of the metros where all these transport investments are concentrated appear to be making little use of it to promote their grassroots development (Ross & Townshend 2018). However, in the recent periods, the increase in the transport expenditure of these metros without the expected economic growth has been a major source of concern to the government (Ross & Townshend 2018). As opposed to the aggregating South Africa’s transport data (see Selamolela 2018), this study investigated the impact of public transportation on the output growth of South Africa using disaggregated data from eight major metros in the country, from 2003 to 2017. The return on transport infrastructure investment in each metro is highlighted.
Unemployment and poverty have continued to unabatedly rise in these metros, thereby affecting their growth rates despite the improvement in transport investment (Mayekiso 2015).
Eight metros are, namely, City of Cape Town (CPT), eThekwini (ETH), Ekurhuleni (EKU), City of Johannesburg (CoJ), Nelson Mandela Bay (NMB), City of Tshwane (CoT), Mangaung (MAN) and Buffalo City (BUFF). For instance, CPT transportation expenditure rose from 120 million Rands in 2007 to about 1.6 billion Rands in 2016 and that of the CoJ rose from 184m Rands to 1.5b Rands within the same period, making these two the highest beneficiaries during this period (National Treasury 2018).
However, other metros appeared to have also been witnessing a similar rise in transport infrastructure investment, for example, ETH 11.8m in 2006 to above 500m Rands in 2016. The underlying rationale is that transportation investment aims at minimising poverty, reducing unemployment and promoting the overall growth and well-being of the metros. Hlotywa and Ndaguba (2017:2) confirmed that ‘transport investment creates the highest number of opportunities and employment in a country’. On the contrary, the growth rate dropped from 5.7% to 4.6% and from 6.3% to 5.2% for COJ and CPT, respectively, between 2007 and 2016 (National Treasury 2018). This similar trend appeared to have been replicated all through the remaining metros. The question then is what has been the impact of investment in transport infrastructure on the growth of these metros?
Various efforts have been made by each metro to enhance their growth and, therefore, their contribution to the South African Gross Domestic Product (GDP); for example, the government’s strategic plan launched in favour of 2014–2019 electoral terms and the ‘Medium Term Strategic Framework’ (MTSF 2014). Both plans have been introduced and applied at the metro level to improve the economic growth and offer optimal utilisation of transport infrastructure such that this will render an improvement in the metros’ output growth (MTSF 2014). The MTSF constitutes the first 5-year execution stage of the national development plan (NDP) (MTSF 2014). It is designed to foster 14 priority outcomes, covering those areas of focus that have been identified in both the NDP and electoral mandates of the government. Areas are those encompassing safety, education, employment and economic growth, infrastructure, social cohesion, nation building, social protection, the public sector, international relations, rural development, local government, environment, human settlements, skills acquisition, development, health and security (MTSF 2014). Regarding the social infrastructures, the strategy comprises doable plans that pave the way for the metros to exploit the fullness of social infrastructures, such as the transport to promote trade and entrepreneurship and, generally, to increase their quota to both provincial and the overall GDP. Specific efforts have been made at the metro level targeted at aiding synergy between economic growth and transport investment. They are
- The Metro Infrastructure Grants − these are designed to eliminate metro infrastructure backlogs. This relates specifically to the backlogs in the metros to ensure the necessary and basic services − portable sanitation, water, community lighting and roads (National Treasury 2014).
- The Bus Rapid Transit System − this is an effective bus-transport system designed to connect diverse portions of provinces and cities (Republic of South Africa 2016).
- The Taxi Recapitalisation Programme − this programme aims at replacing the old model with up-to-date automobile (Mashishi 2010).
- The S’hamba Sonke (‘walking together’) − this is a labour-intensive approach designed as a road-maintenance programme within the provinces of South Africa (Republic of South Africa 2016).
- The Gauteng Freeway Improvement Project − this was inaugurated in 2007 with the aim of easing the mounting congestion along Gauteng routes (Gabriel 2011; Parrock 2015).
Considering all the statistics previously shown, it appears that these efforts are not working.
The literature presents a hybrid view of the impact of transport infrastructure. On the one hand, it is understood the demand for transport space is a derived one, and thus investment may not necessarily lead to growth but is a major contributor (Hesse & Rodrigue 2004). On the other hand, it may be viewed as an important driver for economic growth (Pucher et al. 2007). Lessons from the case of Dubai concur with the latter accessions (Chaudhry 2012; Worku 2013). Cheteni (2013) and Kumo (2012) argued that transport infrastructures alone may not be sufficient to promote growth, without the presence of other socioeconomic factors. In addition, Ross and Townshend (2018) contend that other socioeconomic factors in the metros differ; hence, it is bound to have unevenness in terms of optimum utilisation of transport infrastructures for growth purposes across these metros. This study examines the evidence of the impact of transport infrastructure investment on growth in South Africa’s metros. The rest of the article consists of literature review, methodology, results and discussion and finally conclusions and recommendations.
Literature review
This section is divided into both theoretical and empirical literature, which are discussed as follows.
Theoretical literature (endogenous growth model)
The theory that forms the precursor for the model specification in this study is the endogenous growth model.
The major thrust of the endogenous growth model is the underlying dependence of economic growth on endogenous forces and not external factors (Barro 1990). Endogenous growth theory lays claim to the view that human capital investment, innovation and general knowledge are very important in the growth process and hence contribute in no small measure to economic growth. This model further prioritises externalities that are positive and spillover effect of an economy that is knowledge based with the belief that it will engender economic development. Essentially, parts of the postulations of the endogenous growth model suggest that the long-run growth rate of an economy is determined by public policy measures. For example, subsidies, research and development (R&D) or education as the case may be will increase economic growth. According to the theory, expenditure on an incentive for innovation will have a long-run positive effect on economic growth (Bozkurt 2015; Howitt & Aghion 1998; Inekwe 2015; Romer 1986).
Empirical studies
In developing countries, the impact of transport infrastructure in stimulating growth is not straightforward (Kauzen, Sun & Frank 2020; Meersman & Nazemzadeh 2017). Meersman and Nazemzadeh (2017) noted that the impact of transport infrastructure differs over regions. Transport investment studies have not always been favoured in South Africa. The effect of investment in R&D on the transport infrastructure of South Africa was studied by the researchers (Rust, Van Wyk, Ittmann & Kistan 2008). An increase in expenditure on R&D into transport infrastructure has recently been noted (Rust et al. 2008). Focus on R&D into transport infrastructure is, however, minimal as far as the national agenda is concerned, through a paucity of innovation (Enns 2018). Nevertheless, Negota (2001) noted that the debate amongst the politicians, economists and policymakers about the role of transport infrastructure in improving lives in the third world has been increasing since the mid-1980s. From our survey of the literature, we found that studies have been published on infrastructure investment in general, but only a few have had a focus on transport specifically. We learn from their findings and apply them to transport infrastructure investments.
Kumo (2012) examined the nexus between infrastructure investment and economic growth in South Africa using data from 1960 to 2009. Granger causality test and vector autoregressive (VAR) were applied for the analysis and the results from the study showed a strong bidirectional relationship between the two.
Again, significant long-run ties were found between the two, and it was confirmed that they both have significant influences on themselves. South Africa according to the results can optimise either of the two and obtain positive results on the other. Egert, Kozluk and Sutherland (2009) assessed the impacts of infrastructure network on sustainable economic growth in the Organization for Economic Cooperation and Development nations. Times series analysis (Bayesian VAR) was applied based on the individual country data. The results indicated that transport infrastructure has a direct and significant effect on the economic growth of these countries. Using the panel data analysis, Vlahinić Lenz, Pavlić Skender and Mirković (2018) found the positive impact of transport infrastructure on economic growth in the case of Central and Eastern European Union member states. The results concur with the finding from Maparu and Mazumder (2017), which find a long-run causal relationship between transport infrastructure and economic development in India when using VAR and Vector Error Correction Model (VECM) to the data collected between 1990 and 2011.
The Bayesian VAR confirmed that the highest contribution to the economic growth was by telecommunication and electricity amongst other infrastructure. The effect on Mexico’s output growth of both public and private expenditures on investment was assessed by Nazmi and Ramirez (1997). According to the findings of these authors, public infrastructure investment spending had an important and positive impact on economic growth. The statistical influence of communal investments on output growth was similar to that of the private capital expenditure.
Using data gathered from a wide range of sources on ports, roads, air transports, railways, electricity and telecommunication, Perkins, Fedderke and Luiz (2005) assessed the long-run effect of economic infrastructure in South Africa on output growth. The results concurred with the findings of the F test conducted by Pesaran and Smith (1995). The results revealed that a vital long-run connection may be found between economic growth and economic infrastructure in South Africa. Cheteni (2013) analysed the combined effects of investments in transport infrastructure and transport sector productivity on the output growth of South Africa from 1975 to 2011. Both the VAR analyses and Bayesian VAR were applied to the study. The results showed that domestic-fixed transport investments, and the real inflation (INF) and exchange rates, have a significant influence on economic growth. In the model, when controlled for INF and exchange rate, the results showed an increased influence of infrastructure investment on economic growth. Furthermore, multifactor productivity and lagged value of the GDP also had significant impacts on South African economic growth.
Methodology
Model specification
Several authors (Bosede, Abalaba & Afolabi 2013; Hong, Chu & Wang 2011; Kayode, Babatunde & Abiodun 2013; Mayekiso 2015; Tripathi & Gautam 2010; Xueliang 2008, 2013; Yu et al. 2012) have used the neoclassical growth model to examine the impact of transport infrastructure on output growth. Apart from the model being widely used, it is fitting to our study. Our model is specified below. Apart from public transport expenditure (PTE), S*PTE, L and S, which are public transport expenditure, the interaction of socioeconomic factor expenditure and public transport expenditure, employment at individual metros, socioeconomic factor expenditure, the INF rate is used to capture the macroeconomic policy, whilst K is proxy for the gross fixed asset of the metros:
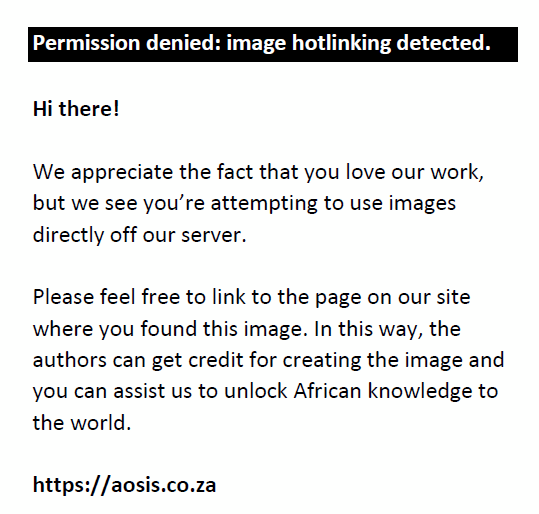
We transform Equation 1 into a logarithmic format. This is to allow us to present influential points in an explicit manner; and, on the other hand, correct skewed variables in the appropriate distribution in the direction of normality (Greene 2003).
Equation 2 into a Cobb–Douglas form:
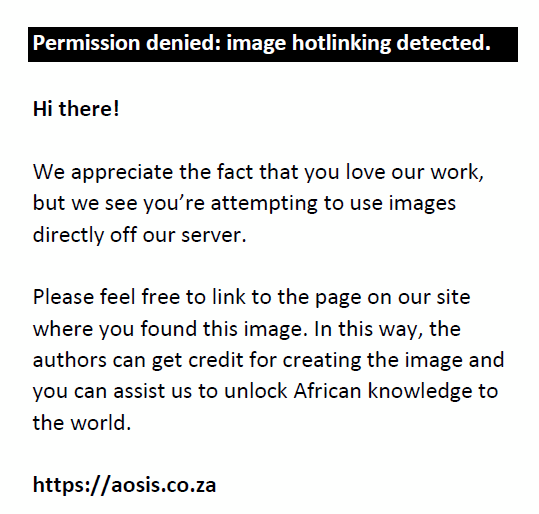
Equation 3 is presented in logarithmic form and transforms it into linear form as follows:
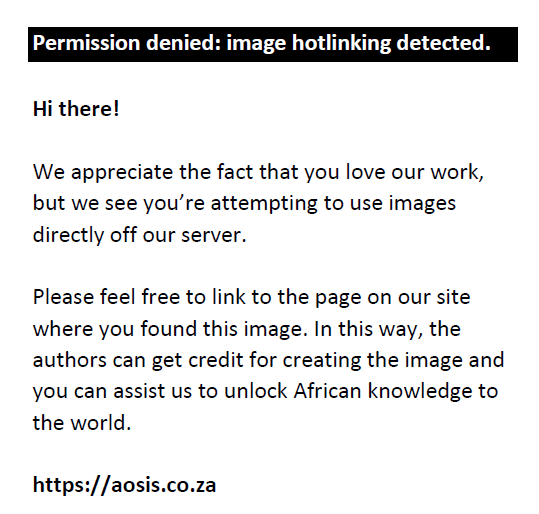
Where Ait is the total factor of productivity. The respective coefficients β0β1β2β3β4and β5 represent the output elasticity or parameters to be estimated with respect to each dependent variable. Table 1 presents variables definition, justification why used and their respective measurement.
TABLE 1: Variables definition, justification and measurement. |
Sources of data
The secondary data were obtained from the IHS Global Insight Regional (IHS Markit 2017), South African Reserve Bank (SARB), South Africa Statistics, National Treasury (2018) on Local Government.
Methd of data analysis
The method of analysis for the study is divided into two because of the objectives, which delve more on exploring the impact of transport infrastructure using the disaggregated approach. Consequently, both panel data and time series approached were applied. The panel data analysis will come first, and this will be followed by time series analysis of individual metros where necessary.
One-ay error component panel regression model
The choice of this estimation procedure is primarily informed by the fact that the study is interested in disaggregating the analysis, which is a clear departure from what has been carried out in the previous studies. The one-way error component panel regression model has a unique feature of allowing the individual effect of each cross-sectional unit examined during the analysis. The equivalent is the fixed effect least square dummy variable (LSDV), which also gives priority to examine the cross-sectional unit effect in the estimated panel model. The fixed effect within regression is also equivalent to the two, but it does not allow the examination of the individual cross-sectional effect. However, it yields similar results in terms of the parameter estimates of the variables included in the model.
The one way error component panel regression model is expressed in Equation 4:
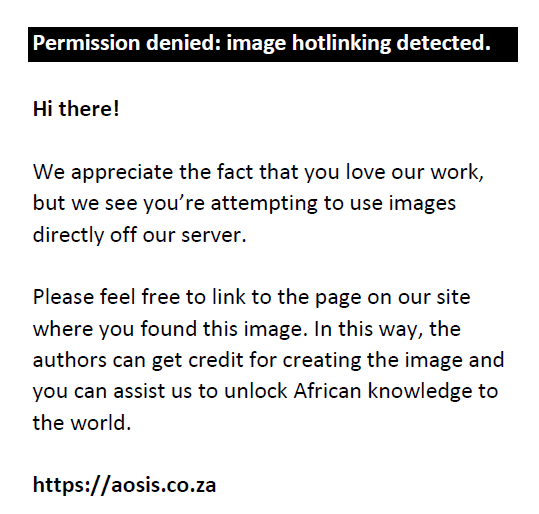
The unique features of the one-way error component panel regression model are that it paves the way for cross-sectional heterogeneity when it comes to the treatment of the error term in the model as expressed by Equation 5:
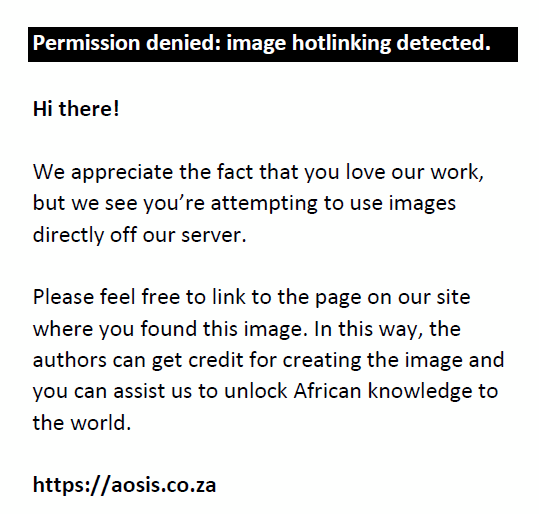
The error term is now modelled in two forms because of the addition of an unobservable component that captures the individual effect, which is time invariant and white noise error term.
However, the individual effect can also be modelled using the fixed effect. This is called the fixed-effect LSDV. This version of the fixed effect is appealing but care must be taken of the numbers of cross-sectional effects to be estimated.
For example (see Equation 6):
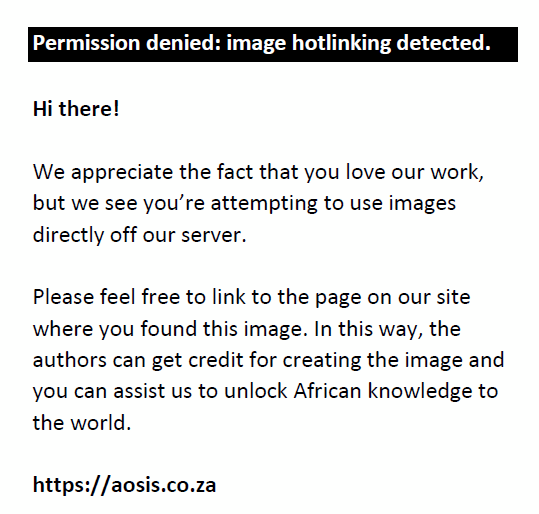
Where K is the number of parameters for the original X-regressor (β’s)
- One is the parameter for the intercept α
- N ‒ 1 is the number of the parameter for the cross-sectional fixed effects, which is also referred to as the omitted cross-sectional effects captured by the intercept. Too many parameters to estimate can undermine the results of the fixed-effect LSDV; hence, the use of the fixed effect within the regression.
Under the regression fixed effects, the cross-sectional effect is still assumed, but they are no longer estimated along with the regression model. The data are demeaned to wipe out the individual effect, and hence only β is estimated, and then the individual effect is calculated.
Autoregressive distribution lag model for each metro
One of the novelties of this study is that it will explore the impacts of transport infrastructure on the growth of each of the eight metros, especially where necessary, by focusing on the time series data from the concerned metro. This is to determine which of the metros is making optimum utilisation of their transport infrastructures to positively influence their growth. This will enable the government to identify the metros where the synergy between transport infrastructure and growth potentials is weak.
However, the procedure for time series analysis of autoregressive distribution lag (ARDL) is very similar to the panel ARDL approach. The major difference is that instead of pooling the data, the analysis will be carried out for each of the eight metros.
Ethical consideration
This study is exempt from ethics review as it relies on public data. Issued by the School of Accounting, Economics and Finance; University of KwaZulu-Natal.
Results and discussions
This study is disaggregated in nature so as to explore individual metros-specific feature regarding the effectiveness of transportation infrastructure on individual metros growth. This will also pave the way for comparative analysis of the results across the metros. The one-way error component panel regression analysis is used to achieve this objective, as explained under methodology. However, other forms of panel estimation such as fixed-effect LSDV and fixed effect within regression will also be used to assess the consistency and robustness of the estimates of the one-way error component panel regression.
However, the analysis starts pre-estimation analysis which is the panel unit root test as all the variables to be included in the estimation need to be stationary.
Panel unit root test
This is the first step under panel estimation. It is required to confirm the suitability of the estimating techniques adopted.
It is evident from Table 2 that all the variables are stationary either at levels or after the first difference.
TABLE 2: Im, Pesaran and Shin IPS, augmented Dickey–Fuller test. |
Variables such as the interaction of social infrastructure expenditure and public transport expenditure and public transport expenditure itself are stationary after the first difference. That is, they are the integration of order I(1) for both IPS and augmented Dickey–Fuller (ADF) unit root test methods. Other variables used in the model apart from these two are all stationary at levels. The result implies that all the variables are suitable for inclusion in the panel model analysis.
Panel data analysis
From the explanation in the methodology, panel data are applied to know the relative effect of public transport expenditure and other output shift factors in the metro on their output growth. The results of the one-way error component panel regression, fixed-effect LSDV and fixed effect regression within are presented in Table 3. They are presented in a single table for the basis of comparison and assessment of consistency in the result.
Table 3 shows the results for the three-panel data analysis used to examine the effect of public transport expenditure on the output growth of the metros in South Africa. The results also show that six of the metros share similarities with regard to the panel presented in the table. This is because the dummy of the two of the metros (BUFF and MAN) are significant whilst the dummy for remaining six are not.
Firstly, the results show that the variables which represent the interactions of social infrastructure expenditure and public transport expenditure have a significant impact on the outputs of the metros. The coefficient from the table of the variable is 0.0196611 and it is significant at 5%. The implication is that the combination of other social infrastructure and public transport expenditures will have positive significant effect on the output of eight metros. Another variable with significant impact on the output growth of the metros is the total expenditure of social infrastructure. Being a derived demand, social infrastructure stimulates the impact of transport infrastructure investment on economic growth. The coefficient is 0.0242986, which shows that social infrastructure has significant positive impact on the output growth of the metros during the period under review. It simply implies that an increase in the expenditure on social infrastructure will bring a significant rise in the output of the metros and vice versa.
Inflation rate is used to capture the effect of macroeconomic policy on the output of the metros. The coefficient of INF in the result is -0.0016917. The value is also significant at 5%. However, the sign is negative, which implies the existence of a significant inverse relationship between the output of the metros and the INF rate.
The implication is that a rise in the level of INF will bring a significant fall to the level of output growth of the metros.
Other variables used in the panel model fail to show significant impact on the output of the metros. However, of them, an important variable out is the public transport expenditure. The result shows that the coefficient is 0.019491. Although the parameter estimates of this variable positive, yet it fails to pass the test of statistical significance at 5%. Therefore, it is concluded that public transport expenditure, in its own right, does not have significant impact on the output of the metros. This may be expected as it is a derived demand. It should, therefore, be considered with other aspects.
The panel result further showed that two of the metros are outliers and this result might not be binding on them because their dummies are significant under both the one-way error component panel regression and fixed-effects LSDV. These metros are BUFF and MAN. The cross-sectional dummy for MAN and BUFF are ‒0.0105391 and 0.0957022, respectively, and they both pass the test of statistical significance at 5%. The implication of this is the likelihood of the results of these two metros, which shows a significant difference from the result of the panel regression presented in Table 3. Furthermore, these two metros can be separately analysed to understand the significant difference in their result from the panel result presented in Table 3.
Diagnostics
Normality test is carried out as a post-estimation test on the panel result to examine the validity and the reliability of the estimated panel model. The result is shown in Figure 1.
 |
FIGURE 1: Normality test for estimated the panel model. |
|
The results from Figure 1 show that the Jarque Bera statistics is 3.548204 and the probability is 0.169636. The implication of this is that the residuals of the panel regression are normally distributed, and hence, no problem of bias in the estimated panel regression. This result adds value to the estimated panel model.
Estimation of the results for Buffalo and Mangaung metros
Following the result from the panel model, it is evident that these two metros are to likely exhibit result that is different from the remaining eight metros. Consequently, ARDL time series regression analysis for the two metros is separately performed to examine this.
Public transport expenditure and output growth of Mangaung metro
The results of the unit root test are presented in Table 4 for MAN metro and ADF test is used.
TABLE 4: Augmented Dickey–Fuller unit root test for Mangaung metro. |
Unit root test result for MAN metro as presented in Table 4 indicates that the variables’ order of integration ranges between order 1 and order 0. The log of social infrastructure expenditure, the log of the interaction between public transport expenditure and social infrastructure expenditure as well as the inflation rate are I(0) which means that they are stationary at their level. The other variables in the model, namely, the growth rate of the metro, labour, and capital are all I(1), which means that they are stationary at first difference. Consequently, the ARDL estimation can be applied. However, first the cointegration test is carried out before the estimation of the ARDL regression.
Autoregressive distribution lag regression estimation for Mangaung
Table 5 summarises the impacts of transport infrastructure on the output growth of MAN metro.
TABLE 5: Autoregressive distribution lag short- and long-run results for Mangaung. |
The result shown in Table 5 is an indication that many of the variables have significant impact on the output growth of the MAN metro.
The results of the MAN metro indicate that many of the variables used as proxy for transport infrastructure have significant impact on the growth of the metro. The social infrastructure expenditure, the interaction of public infrastructure expenditure, and the total expenditure on social infrastructure have significant impacts on the growth of the metro in the short run. However, only the interaction of public infrastructure expenditure that have the impact in the long run. This further justifies the fact that combination of the social infrastructure and public transport expenditure significantly influences the growth of the metro.
Amongst other control variables, labour and capital show significant impact on the growth of the MAN metro during the period under review. In addition, the macroeconomic variable used in the model, that is INF rate, also show significant impact on the growth of the metro. The error correction term coefficient is -0.120434. The coefficient is negative and statistically significant at 5%, which is an indication that the adjustment process is in the right direction and also indicates that when there are disequilibrium, forces that will restore the equilibrium will set in.
Mangaung diagnostic tests
Three tests are conducted to assess the validity of the ARDL regression results, namely, the test of serial correlation, the heteroscedasticity test and the normality test. Their results are presented as follows.
The null hypothesis in Table 6 is that no serial correlation is involved. Considering the probability of the F statistics, a noticeable indication no serial correlation problem in the estimated ARDL model exists as the null hypothesis is accepted.
TABLE 6: Serial correlation test for Mangaung. |
TABLE 7: Test for heteroschedaticity for Mangaung. |
Similarly, the null hypothesis is ‘no heteroscedasticity’ as all the probabilities of the test statistics are greater than 5%, indicating that the null hypothesis is accepted. In this context, it can be concluded that no heteroscedasticity is observed.
The Jarque Bera statistics is used to assess the normality of the estimated ARDL model and the results are presented in Figure 2. The Jarque Bera probability is 0.099628, which shows that the model is normally distributed. The implication is that the inferences drawn from the ARDL estimated model are valid.
Public transport infrastructure and output growth of Buffalo City
The ADF test is applied to ascertain the order of integration of each variable in the model.
The results of the unit root test indicate that two of the variables are stationary, whilst others are non-stationary (see Table 8). However, the ones that are non-stationary eventually become stationary after the first deference, thereby they are integrated with order I(1). Only INF rate and social expenditure infrastructure are stationary at levels. The findings under the unit root test show that ARDL approach conditions are satisfied. The next is the integration test.
TABLE 8: Augmented Dickey–Fuller unit root test for Buffalo metro. |
Buffalo autoregressive distribution lag regression estimation
The time series estimation using the ARDL approach for BUFF metros is explained under this section.
The results from the BUFF metro are presented in Table 9, which show that all the variables virtually have significant impacts on the growth of BUFF. Public transport expenditure, social infrastructure expenditures and the interaction between the two all have significant impact on the growth rate of BUFF metro. For instance, the coefficients are 0.034802, 0.034105 and 0.025074, respectively, and they are all individually significant at 5%.
TABLE 9: Autoregressive distribution lag short- and long-run results for Buffalo. |
Other variables, such as labour which is proxy for total labour cost and capital which is proxy for the value of fixed assets, all have significant impact on the growth of BUFF metro during the period under review. However, INF failed to show significant impact on the output of the metro. These may be because of South Africa’s INF target.
Apart from the fact that there is a long-run relationship amongst the variables, all the variables that have significant impact in the short-run are also significant in the long-run. It implies that public transport expenditure, social infrastructure expenditure and other variables, all have sustainable impact on the growth of the BUFF metro.
The error correction model coefficient is negative and significant. The value of the error correction is ‒0.123417 and it is significant at 1%. This is an implication that the adjustment to equilibrium in BUFF is in the right direction; and whenever there a disequilibrium, forces that restore the equilibrium will set in.
Buffalo diagnostic tests
Three tests are conducted to assess the validity of the ARDL regression results, namely, the test of serial correlation, the heteroscedasticity test and the normality test. Their results are presented as follows.
The null hypothesis in Table 10 is that there is no serial correlation. Considering the probability of the F statistics, there is a noticeable indication that there is no serial correlation problem in the estimated ARDL model as the null hypothesis is accepted.
TABLE 10: Buffalo metro serial correlation test. |
Similarly, the null hypothesis is ‘no heteroscedasticity’ as all the probabilities of the test statistics are greater than 5%, indicating that the null hypothesis is accepted. Table 11 confirms that, in this case, it can be concluded that there is no heteroscedasticity.
TABLE 11: Test for heteroschedaticity for Buffalo metro. |
The Jarque Bera statistics is used to assess the normality of the estimated ARDL model, and the results are presented in Figure 3. The Jarque Bera probability is 61.31166, which shows that the model is not normally distributed. Notwithstanding, both the heteroscedasticity and serial correlation tests are OK; and hence, it reduces the negative implication of the normality test.
Conclusions
Revelation from the study indicates that in big metros, public transport expenditure alone failed to influence their output growth until it is combined with other social infrastructure expenditures. The result underscores the importance of other social infrastructures in the metros as important shift factors of output. It is confirmed from the result that the combination of transport infrastructures, health and education amongst others will jointly and significantly influence the output of these metros. The big metros are CPT, CoJ, EKU, ETH, NMB and CoT. The implication is that transport infrastructure alone cannot significantly promote the output growth of these six metros unless it is combined with other social infrastructures.
The study has also shown a significant difference in the result of the studies that used aggregated approach, and this study that used disaggregated approach as the conclusion from those studies run contrary to what was obtained in some of the metros investigated in this study. This further justified the split of the analysis to individual metros rather than studying the effect of transport expenditure on the entire output growth of South Africa as it was carried out in some previous studies.
It is also an important conclusion from the study that CPT, CoJ, EKU, ETH, NMB and CoT have the most similar results as presented by the panel analysis, where all the data from the eight metros are pooled. Therefore, it is important to note that conclusions from the panel data would have been only valid for these six metros, leaving the other two remaining metros, namely, MAN and BUFF. Generally, it can be concluded from the study that public transport infrastructure impact on output generation in these metros is highly sensitive to the size of the metros. This may also be associated with the population propensity to spend and infrastructure spend per population within the metros. For which this is an area for future research. This is evident from the findings which have shown that the smaller metros outperformed the big metros in terms of the significant impact of public transport expenditure on their outputs. There are more economies of scale that can be achieved if investments may be directed to smaller metros than bigger metros.
Acknowledgements
We would like to acknowledge the Macroeconomics Research Unit of the University of KwaZulu-Natal for the support and guidance.
Competing interests
The authors have declared that no competing interest exist.
Authors’ contributions
L.K.H. and S.G. contributed equally to this work.
Funding information
This research received no specific grant from any funding agency in the public, commercial or not-for-profit sectors.
Data availability
The authors confirm that the data supporting the findings of this study are available within the article.
Disclaimer
The views and opinions expressed in this article are those of the authors and do not necessarily reflect the official policy or position of any affiliated agency of the authors.
References
Barro, R.J., 1990, ‘Government spending in a simple model of endogenous growth’, Journal of Political Economy 98(5, Part 2), S103–S125. https://doi.org/10.1086/261726
Bosede, A., Abalaba, B. & Afolabi, D., 2013, ‘Transport infrastructure improvement and economic growth in Nigeria’, International Journal of Humanities and Social Science Invention 2(8), 26–31.
Bozkurt, C., 2015, ‘R&D expenditures and economic growth relationship in Turkey’, International Journal of Economics and Financial Issues 5(1), 188.
Chaudhry, G.A., 2012, ‘Evolution of the transportation system in Dubai’, Network Industries Quarterly 14(1), 7–11.
Chen, Y., Salike, N., Luan, F. & He, M., 2016, ‘Heterogeneous effects of inter-and intra-city transportation infrastructure on economic growth: Evidence from Chinese cities’, Cambridge Journal of Regions, Economy and Society 9(3), 571–587. https://doi.org/10.1093/cjres/rsw019
Cheteni, P., 2013, ‘Transport infrastructure investment and transport sector productivity on economic growth in South Africa (1975–2011)’, Mediterranean Journal of Social Sciences 4(13), 761. https://doi.org/10.5901/mjss.2013.v4n13p761
Égert, B., Kozluk, T.J. & Sutherland, D., 2009, Infrastructure and growth: Empirical evidence, OECD Publishing, Paris.
Enns, C., 2018, ‘Mobilising research on Africa’s development corridors’, Geoforum 88, 105–108. https://doi.org/10.1016/j.geoforum.2017.11.017
Fedderke, J.W. & Bogetic, Z., 2009, ‘Infrastructure and growth in South Africa: Direct and indirect productivity impacts of 19 infrastructure measures’, World Development 37(15), 22–39.
Gabriel, C.C., 2011, ‘An assessment of the South African government’s Gauteng freeway improvement project (GFIP) toll road strategy’, PhD thesis, University of South Africa, Pretoria.
Greene, W., 2003, Econometric analysis, 5th edn., Pearson Education, Upper Saddle River, NJ.
Hesse, M. & Rodrigue, J.P., 2004, ‘The transport geography of logistics and freight distribution’, Journal of Transport Geography 12(3), 171–184. https://doi.org/10.1016/j.jtrangeo.2003.12.004
Hlotywa, A. & Ndaguba, E.A., 2017, ‘Assessing the impact of road transport infrastructure investment on economic development in South Africa’, Journal of Transport and Supply Chain Management 11(1), 1–12. https://doi.org/10.4102/jtscm.v11i0.324
Hong, J., Chu, Z. & Wang, Q., 2011, ‘Transport infrastructure and regional economic growth: Evidence from China’, Transportation 38(5), 737–752. https://doi.org/10.1007/s11116-011-9349-6
Howitt, P. & Aghion, P., 1998, ‘Capital accumulation and innovation as complementary factors in long-run growth’, Journal of Economic Growth 3(2), 111–130. https://doi.org/10.1023/A:1009769717601
IHS Markit, 2017, IHS global insight regional, viewed 15 July 2018, from https://www.ihsmarkit.co.za/Products/ReX/.
Inekwe, J.N., 2015, ‘The contribution of R&D expenditure to economic growth in developing economies’, Social Indicators Research 124(3), 727–745. https://doi.org/10.1007/s11205-014-0807-3
Kauzen, R., Sun, B. & Frank, H., 2020, ‘Does transport infrastructure have an impact on economic growth? Observed fact from Tanzania’, in Proceedings of the 2020 4th International conference on management engineering, software engineering and service sciences, Association for Computing Machinery, Wuhan, China, January 17–19, 2020, pp. 255–258.
Kayode, O., Babatunde, O. & Abiodun, F., 2013, ‘An empirical analysis of transport infrastructure investment and economic growth in Nigeria’, Social Sciences 2(6), 179–188. https://doi.org/10.11648/j.ss.20130206.12
Kumo, W.L., 2012, ‘Infrastructure investment and economic growth in South Africa: A granger causality analysis’, African development bank group working paper series, 160, African Development Bank Group, Tunis.
Maparu, T.S. & Mazumder, T.N., 2017, ‘Transport infrastructure, economic development and urbanization in India (1990–2011): Is there any causal relationship?’, Transportation Research Part A: Policy and Practice 100, 319–336. https://doi.org/10.1016/j.tra.2017.04.033
Mashishi, S.T.D., 2010, ‘The taxi recapitalisation programme: Some perceptions of the taxi associations in Temba’, PhD thesis, University of Johannesburg, Johannesburg.
Mayekiso, S., 2015, ‘The impact of transport infrastructure investment on unemployment in South Africa’, Masters dissertation, University of Fort Hare, Alice.
Medium-Term Strategic Framework (MTSF), 2014, Medium-Term Strategic Framework 2014–2019, Department Planning, Monitoring and Evaluation, Pretoria.
Meersman, H. & Nazemzadeh, M., 2017, ‘The contribution of transport infrastructure to economic activity: The case of Belgium’, Case Studies on Transport Policy 5(2), 316–324. https://doi.org/10.1016/j.cstp.2017.03.009
Morgenroth, E., 2014, ‘The regional development impacts of transport infrastructure: A literature review and policy implications’, Research Series, RePEc: Esr: Resser: bkmnext278, Department Of Transport, Tourism and Sport, Dublin.
National Treasury, 2014, Review of local government infrastructure grants, National Treasury, Pretoria.
National Treasury, 2018, Vulekamali webisite, viewed 14 June 2018, from https://vulekamali.gov.za/.
Nazmi, N. & Ramirez, M.D., 1997, ‘Public and private investment and economic growth in Mexico’, Contemporary Economic Policy 15(1), 65–75. https://doi.org/10.1111/j.1465-7287.1997.tb00455.x
Negota, G.M., 2001, The impact of transport investment on infrastructure and economic development: The debate, Southern African Transport Conference (SATC), Pretoria.
Parrock, P., 2015, ‘Mega project analysis: A case study of the Gauteng freeway improvement project’, Doctoral dissertation, Stellenbosch University, Stellenbosch.
Perkins, P., Fedderke, J. & Luiz, J., 2005, ‘An analysis of economic infrastructure investment in South Africa’, South African Journal of Economics 73(2), 211–228. https://doi.org/10.1111/j.1813-6982.2005.00014.x
Pesaran, M.H. & Smith, R., 1995, ‘Estimating long-run relationships from dynamic heterogeneous panels’, Journal of Econometrics 68(1), 79–113. https://doi.org/10.1016/0304-4076(94)01644-F
Pucher, J., Peng, Z.R., Mittal, N., Zhu, Y. & Korattyswaroopam, N., 2007, ‘Urban transport trends and policies in China and India: Impacts of rapid economic growth’, Transport Reviews 27(4), 379–410. https://doi.org/10.1080/01441640601089988
Republic of South Africa, 2016, South Africa Yearbook 2015/16, viewed 21 April 2017, from https://www.gcis.gov.za/sites/default/files/docs/resourcecentre/yearbook/SAYB1516.pdf
Rust, F., Van Wyk, L., Ittmann, H.W. & Kistan, K., 2008, The role of R&D in transport infrastructure in South Africa, Southern African Transport Conference (SATC), Pretoria.
Romer, P.M., 1986, ‘Increasing returns and long-run growth’, Journal of Political Economy 94(5), 1002–1037. https://doi.org/10.1086/261420
Ross, D. & Townshend, M., 2018, ‘The economic importance of an optimal road investment policy in South Africa’, In 37th Annual Southern African Transport Conference (SATC 2018), Pretoria, South Africa, July 09–12, 2018, pp. 329–339, viewed n.d., from https://www.satc.org.za/assets/2a_townshend.pdf.
Rust, F., Van Wyk, L., Ittmann, H.W. & Kistan, K., 2008, ‘The role of R&D in transport infrastructure in South Africa’, 27th Southern African Transport Conference (SATC), Pretoria, South Africa, July 07–11, 2008, pp. 1–14.
Selamolela, N., 2018, ‘The impact of transport infrastructure in the economic growth of South Africa’, PhD thesis, University of Cape Town, Cape Town.
Skorobogatova, O. & Kuzmina-Merlino, I., 2017, ‘Transport infrastructure development performance’, Procedia Engineering 178, 319–329. https://doi.org/10.1016/j.proeng.2017.01.056
Tong, T., Yu, E. & Roberts, R.K., 2014, ‘Dynamics of transport infrastructure, exports and economic growth in the United States’, Journal of the Transportation Research Forum 53(1), 65–81.
Tripathi, S. & Gautam, V., 2010, ‘Road transport infrastructure and economic growth in India’, Journal of Infrastructure Development 2(2), 135–151. https://doi.org/10.1177/097493061100200204
Vlahinić Lenz, N., Pavlić Skender, H. & Mirković, P.A., 2018, ‘The macroeconomic effects of transport infrastructure on economic growth: The case of central and eastern EU member states’, Economic Research – Ekonomska istraživanja 31(1), 1953–1964. https://doi.org/10.1080/1331677X.2018.1523740
Worku, G.B., 2013, ‘Demand for improved public transport services in the UAE: A contingent valuation study in Dubai’, International Journal of Business and Management 8(10), 108. https://doi.org/10.5539/ijbm.v8n10p108
Xueliang, Z., 2008, ‘Transport infrastructure, spatial spillover and economic growth: Evidence from China’, Frontiers of Economics in China 3(4), 585–597. https://doi.org/10.1007/s11459-008-0029-1
Xueliang, Z., 2013, ‘Has transport infrastructure promoted regional economic growth? – With an analysis of the spatial spillover effects of transport infrastructure’, Social Sciences in China 34(2), 24–47. https://doi.org/10.1080/02529203.2013.787222
Yu, N., De Jong, M., Storm, S. & Mi, J., 2012, ‘The growth impact of transport infrastructure investment: A regional analysis for China (1978–2008)’, Policy and Society 31(1), 25–38. https://doi.org/10.1016/j.polsoc.2012.01.004
|