Abstract
Background: The economic deregulation of the airline industry in South Africa in 1991 was a landmark event and brought about various changes in the air transport market, both locally and internationally. One important after-effect of deregulation was the entry of low-cost carriers (LCCs) in 2001, which increased competition in the market and offered passengers the freedom to choose between full-cost carriers and LCCs. It is generally accepted that LCCs have been very successful across the globe, and the main reason for this lies in their simplified lower cost business models. One way of achieving lower costs is for LCCs to operate from secondary or alternative airports. This trend is observed in most regions of the world. In South Africa, and more specifically the Gauteng province, Lanseria International Airport is considered as an alternative airport to OR Tambo International Airport (the main international airport of South Africa and located about 30 km east of the Johannesburg Central Business District [CBD]). Currently, two LCCs operate from this airport with a third LCC airline indicating that it will shortly begin operations from this airport.
Objectives: The research presented here reflects on the aspects passengers consider when selecting a secondary airport for their travel needs. It also compares the research findings of passenger attributes when choosing Lanseria Airport as a secondary airport in 2010 to a similar study in 2013 after another LCC commenced operations from the airport.
Method: In this exploratory research a face-to-face survey was used as the quantitative data collection method in order to identify the factors that influenced passengers’ airport choice decisions at Lanseria International Airport.
Results: From this research it emerged that when airports in a metropolitan area are close to one another, one of the main considerations for passengers is access time when selecting an airport. Even after a second LCC started operating from Lanseria International Airport, the attributes passengers regard as important in their decision to fly from the airport remained unchanged.
Conclusion: The aim of the research is to gain a deeper understanding of the factors involved in secondary airport selection and, building on this knowledge, to assist airport owners and managers in positioning their airports in a multi-airport competitive environment. Similarly, the findings of the research could assist airlines in their decision-making process to operate from secondary airports
Introduction
Air travel has generally been regarded as the fastest growing mode of transport during the 20th century (Wensveen 2011). Two of the main reasons for the ongoing growth in air travel during the latter half of the previous century were, firstly, the introduction of low-cost airlines and, secondly, the use of secondary airports,1 as opposed to primary airports, by these airlines (Vasigh, Fleming & Tacker 2008). According to Barbot (2006), low-cost airlines and their use of secondary airports are closely related. The interrelationship between these two concepts is important. It is unlikely that the international growth of low-cost airlines could have taken place without recognising the role of secondary airports (De Neufville 2005). Reasons for this are not only linked to the business model of low-cost airlines but also relate to the airport choice factors considered by low-cost airlines (Warnock-Smith & Potter 2005), which can be summarised as follows:
- lower airport charges
- quicker turnarounds
- modest terminals
- speedy check-in facilities
- good passenger facilities
- ease of access
- less congestion compared to major airports or hubs.
Following airline deregulation in the United States, and liberalisation of the European markets, low-cost airlines emerged and have had a significant impact on the airline industry (De Wit & Zuidberg 2012). In the US market, Southwest Airlines has had a major impact on the airline market and has been the pioneering low-cost airline in this region (Bennet & Craun 1993). In Europe the number of low-cost airlines increased significantly after the first flight of Ryanair commenced in 1986 (Barrett 2004).
In South Africa, the domestic deregulation of the air transport industry took place in 1991. This brought about new prospects for airlines to enter the market, resulting in changes to the role of the established airlines at that time (Smith 1998). Some of the consequences of airline deregulation in South Africa were service innovations such as an increase in frequent-flyer packages, a variety of fare classes, existing airlines expanding into the wider domestic market (Smith 1998), new airlines being established, and eventually the introduction of low-cost carriers (LCCs) in 2001 (Luke & Walters 2013).
Passengers have benefited the most from air transport deregulation and liberalisation together with the development of LCCs. Some benefits include:
- Increased choice: After deregulation, barriers for new entrants into the air transport market were removed, which increased the number of airlines. This resulted in passengers no longer being restricted to national carriers but having the freedom to choose between various airlines. Routes were served by more airlines than before as a result of the removal of air service agreements that restricted traffic on routes. Passengers at the origin and destination of a route had a greater choice of schedules, frequencies and airports to fly from.
- Lower fares: Increased competition in the air transport market and the growth of LCCs led to a decrease in airfares. Traditional airlines are in many cases forced by competitive pressure from LCCs to lower their fares to retain their market share on routes serviced by both types of airlines. Research indicates that many passengers flying with LCCs ‘would not have travelled by air had it not been for low fares’ (European Low Fares Airline Association [ELFAA] 2004:16).
Passenger airport choice factors
Studying passenger airport choice determinants may be of great value to airport managers as it can assist with determining passengers’ demand at the airport. It can inform airport planners from which catchment area an airport is likely to attract passengers (Windle & Dresner 1995).
Prior to the emergence of the use of secondary airports by LCCs, passengers mainly had to fly from the primary or core airport in a specific region. With the increase in the number of destinations served by LCCs, passengers can now choose to fly from an alternative airport in relatively the same area as the primary airport. In these multi-airport cities or regions, airport choice becomes an essential air travel related decision (Jiangtao 2009). Wide-ranging research has been done on airport choice models from a passenger perspective, especially in urban areas with multiple airports available to passengers (Barbot 2009; Marucci & Gatta 2011; Skinner 1976; Windle & Dresner 1995; Zhang & Xie 2005). Various other researchers have studied airport choice to explore the passengers’ airport choice determinants (Jiangtao 2009). These studies included different airports and/or different classes of passengers. Harvey (1987) researched passenger airport choice with specific reference to airport access modes and found that in the San Francisco Bay Area, travel time and travel costs are significant elements in choosing an airport. Innes and Doucet (1990) established that the type of aircraft and flying time differences are considered as vital aspects influencing a passenger’s choice of airport. Many studies have shown that passengers will actually choose the airport that is located closest to them (Goedegebuure 2010). A study conducted by Windle and Dresner (1995) in the Washington DC and Baltimore areas indicated that:
- Time taken to access an airport and frequencies of flights from airports were vital elements of airport choice.
- Passenger experience at an airport is a rather significant factor in airport choice.
- Where there are competing airports, the importance of airport access time declined, but flight frequencies became more important.
In all the airport choice studies mentioned above, two variables consistently emerge as significant:
- travel access time
- flight frequencies.
Barbot (2009) suggests that when passengers have to choose between two airports, they actually consider the grouping of airports and airlines and not the airport alone. Airport choice is a complex decision in a multi-airport region and can be influenced by various factors such as (Marucci & Gatta 2011):
- capacity expansion
- parking policies
- ground transportation improvements
- ground service efficiency
- connectivity.
According to Zhang and Xie (2005), when airports in a specific metropolitan area are located close to one another, the only major difference will be airport access time and flight frequency.
A study conducted by Pels, Nijkamp and Rietveld (n.d.) found that travellers make a sequential decision; in other words, they will choose the departure airport first, and the airline second. Hess (2010) determined that passengers tend to dislike larger airports due to the professed stress of using such airports and also the possibility of delays. Passengers tended to favour airports closer to their homes. Although passengers seem to dislike the larger airports, they would still choose flight options from such an airport owing to the perceived higher levels of service, such as backup options in case of flight cancellation.
Goedegebuure (2010) found that the following are the most important factors in passengers’ airport choice:
- Cost: Air travel cost consists of various components including the airfare itself, parking costs, as well as cost of getting to the airport.
- Flight characteristics: These include aspects such as flight connection quality, flight schedules and aircraft type (jet aircraft vs. non-jet aircraft).
- Travel time: Important elements include distance to the airport and accessibility of the airport.
- Airport facilities: Elements include the number and quality of facilities at airports, such as number of shops, number and quality of restaurants as well as number and quality of lounges.
- Time between arrival at airport and boarding: Variables that determine the time a passenger spends at the airport before boarding the aircraft include parking facilities, check-in time, security issues and distance from check-in counter to boarding gate.
Some characteristics of the domestic aviation market in South Africa
The busiest domestic airline network in Africa can be found in South Africa between Johannesburg (located in the Gauteng province), Cape Town and Durban, and is termed the ‘Golden Triangle’ (News24 2013). The LCCs in South Africa also focus their operations on the so-called Golden Triangle (Luke & Walters 2013). The Johannesburg–Cape Town route was identified as one of the world’s ten busiest flight routes measured by passenger volume, carrying 4.4 million passengers in 2012, from a survey conducted by Amadeus Air Traffic Travel Intelligence Solution. (News24 2013). South African Airways used to dominate the Golden Triangle in the past but has lost market share on these high-density domestic routes, due to the entry of LCCs (Luke & Walters 2013).
In the Gauteng province, the smallest of nine provinces by surface area and considered to be the industrial hub of SA, there are two airports that are used by scheduled airlines to provide domestic passenger services within South Africa.2 The first airport is OR Tambo International Airport (ORTIA), situated to the east of Johannesburg, and is regarded as the air transport hub of southern Africa. Over 19 million passengers make use of this airport each year and comprise international, regional and domestic passengers (Airports Company South Africa [ACSA] 2015).
The second airport is Lanseria International Airport (LIA or Lanseria), and is regarded as a secondary airport to ORTIA. The airport is conveniently situated close to Sandton, a business hub in Gauteng. The downtown areas of the cities of Johannesburg and Pretoria are both approximately 50 km away. The airport experienced low volumes of scheduled passenger travel until 2006, when Kulula commenced operations from there (in addition to its services from ORTIA which commenced in 2001) as a low-cost airline. Since then, Kulula has gradually grown its passenger volumes from Lanseria (Leitch 2011). In June 2012, a second LCC, Mango Airlines, began operations from this airport (Mokgatain Financial Mail 2012). Currently Kulula and Mango Airlines operate scheduled, daily flights from Lanseria Airport to Cape Town and Durban. Lanseria Airport also supports various charter services to a variety of destinations and is a major regional business aircraft and maintenance hub.
At the time of the study there were three LCCs that served the domestic passenger market, namely Kulula, Mango Airlines and 1Time. 1Time operations were terminated in 2012 (News24 2012) and the airline did not offer services from Lanseria during the study period. FlySafair commenced operations in October 2014 (FlySafair 2015). The airline intends to commence operations from Lanseria in September 2016 (IOL 2016). It was therefore not included in the study.
Since passengers currently have a choice between ORTIA and Lanseria airports when flying to either Cape Town or Durban, and since the airlines operating from Lanseria also operate from ORTIA (serving the same destinations), it is opportune to determine the reasons for airport selection from a passenger’s point of view. The Institute of Transport and Logistics Studies (ITLS) (Africa) conducted a study in 2010 to determine passenger choice decisions at Lanseria when only one low-cost airline, Kulula, operated from there (Heyns & Carstens 2011). Now that another low-cost airline, Mango Airlines, also offers flights from the airport, it is appropriate to investigate passengers’ choices again to establish if the factors influencing the passengers’ choice of airport have changed because of the entrance of Mango.
Research objectives
The purpose of this research is to determine why Lanseria International Airport is a preferred airport for users and, secondly, to determine if passengers’ airport choice attributes differ after the entry of a second scheduled domestic low-cost airline that operates from the airport.
Research methodology
In this study, comparisons will be drawn between the results of this study, conducted in 2013, and that of a similar study conducted in 2010 by the ITLS (Africa), thereby addressing the primary objective of the research – namely, to determine the reasons passengers prefer to fly to and from Lanseria Airport.
Primary and secondary data will be analysed. The primary data to be analysed consist of responses from a passenger survey conducted at Lanseria Airport in 2013. This 2013 survey followed the first survey by the ITLS (Africa) which was conducted in 2010.
Secondary data to be analysed are from a similar survey conducted in 2010 by the ITLS (Africa) and statistically analysed by Heyns and Carstens (2011). The latter survey consisted of a questionnaire completed by a random sample of departing passengers at Lanseria International Airport and was used to determine the main passenger airport choice attributes. At the time of the survey, only Kulula offered scheduled domestic services from Lanseria Airport to Cape Town International Airport and to the then Durban International Airport (DIA) (in May 2010 the DIA operations were moved to the new King Shaka International Airport north of Durban after the closure of DIA).
The 2013 survey, similar to that of the ITLS (Africa) of 2010, was conducted again at Lanseria International Airport as the context at this airport had changed substantially with the entry of Mango Airlines. Therefore, passengers travelling on both Mango Airlines (who started offering scheduled domestic flights from Lanseria International Airport in 2012) and Kulula were interviewed in the 2013 survey. This survey followed the same survey methodology used in the 2010 survey.
The sample in the 2010 survey used stratified random sampling to be representative of the weekly departure schedule of Kulula from Lanseria Airport (Heyns & Carstens 2011). Similarly, in 2013, a stratified random sample of passengers was selected to be representative of the weekly departure schedules of both Kulula and Mango Airlines from Lanseria International Airport. Both surveys were paper-based surveys administered by trained interviewers.
For the 2010 survey, a random sample of 210 departing passengers was surveyed over the period of 29 September – 04 October 2010 (Heyns & Carstens 2011). In the 2013 survey, 308 randomly selected passengers were surveyed during the first week of February 2013.
For both surveys the sample sizes were verified by STATCON (Statistical Consultation Service)3 as being adequate for the type of survey undertaken.
Results
Survey conducted in 2010
The purpose of the research conducted in 2010 was to identify the underlying factors which influenced passenger airport choice at Lanseria Airport in the Gauteng area in South Africa.
The 2010 questionnaire (ITLS 2010) comprised 18 attributes related to the passengers, airline offerings and the airport, such as:
- demographics
- price
- service
- convenience.
The respondents were asked to complete a forced 4-point Likert-type scale to rank the influence of each attribute on their decision to use Lanseria International Airport. The scale included the following range of choices: (1) to no extent (2) to a small extent, (3) to a moderate extent and (4) to a large extent (Heyns & Carstens 2011).
The survey included a systematic random sample of 210 departing passengers at Lanseria Airport. The random selection of passengers was systematically structured to be representative of the weekly departure schedule of the low-cost airline, Kulula (Heyns & Carstens 2011). The respondents were all departing passengers at the airport.
Survey conducted in 2013
The purpose of the study conducted in 2013 was to identify the underlying factors which influenced passenger airport choice decisions at Lanseria Airport following the entry of a second low-cost airline. The information was obtained through a survey of departing passengers at the airport. The 2013 questionnaire comprised 19 attributes relating to the following aspects of the passengers, airline offerings and airport:
- demographics
- price
- service
- convenience.
Respondents were asked to complete a forced 4-point Likert-type scale to rank the influence of each attribute on their decision to use Lanseria Airport. The scale included the following range of choices: (1) to no extent, (2) to a small extent, (3) to a moderate extent and (4) to a large extent. In utilising this choice method, the option of undecided or neutral is not available.
The survey included a systematic random sample of 308 departing passengers at the airport. The random selection of passengers was systematically structured to be representative of the weekly departure schedule of the two low-cost airlines, namely Kulula and Mango Airlines.
Results of the survey
The majority of the respondents (70.7%) used Kulula to travel in 2013 as opposed to Mango Airlines, as depicted in Figure 1. The reason for this is that Kulula offers more flights per week (Kulula 2013) than Mango Airlines, and the latter only offers flights to Cape Town and not to Durban (Mango Airlines 2013). Mango Airlines only commenced flights to Durban in October 2015 (Traveller 24 2015b). In 2010, only Kulula offered flights from Lanseria International Airport (Heyns & Carstens 2011).
Residential distribution of respondents
It is evident from Figure 2 that in 2013 respondents mainly resided in Gauteng (59.7%), followed by the Western Cape (23.4%) and KwaZulu-Natal (12.3%).
The same distribution by province of residence was evident in the 2010 survey (ITLS 2010), as indicated in Figure 2.
Zhang and Xie (2005) found that when airports in a metropolitan area are located close to one another, one of the main factors passengers consider when choosing an airport is access time. Lanseria International Airport is easily accessible for air passengers residing in the northern, central and western suburbs of Gauteng.
To test the theory that passengers choose the airport closest to them, the questionnaire information of both surveys was used to plot the residential (suburb) postal codes of respondents on a map of Gauteng. In order to determine the geographical location of each suburb on the map, estimated x- and y-values were given to each suburb. The number of respondents per suburb was presented as a percentage of the total respondents who reside in Gauteng. These percentages are indicated by the green dots in Figure 3 (2010) and Figure 4 (2013).
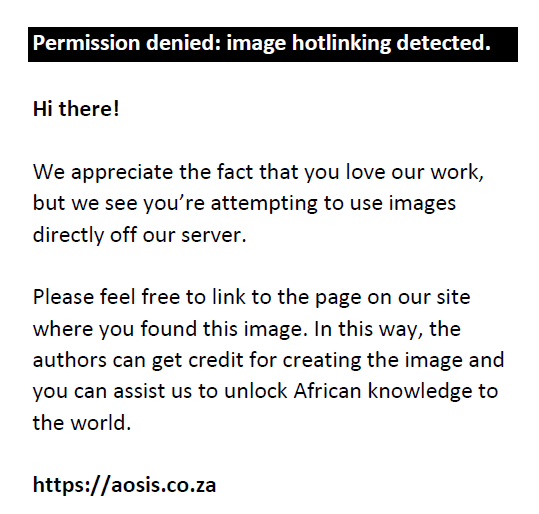 |
FIGURE 3: Gauteng respondents’ residential distribution (2010). |
|
 |
FIGURE 4: Gauteng respondents’ residential distribution (2013). |
|
According to the survey conducted in 2010 (ITLS 2010), the passengers using Lanseria Airport generally resided in the northern, western and central regions of Gauteng as indicated by the green dots in Figure 3.
In 2013, the respondents using Lanseria International Airport similarly resided in the northern, western and central regions of Gauteng, as indicated by the green dots on Figure 4.
Both figures indicate the same passenger distribution, confirming Hess (2010) who postulates that passengers tend to favour airports closer to their homes and tend to dislike larger airports. Passengers choose to fly from an airport that is located closer to them because travel time to the airport will be lower (Barbot 2006).
Factor analysis
In the section that follows the results of the 2013 findings are compared to the published findings of the 2010 ITLS (Africa) study (Heyns & Carstens 2011).
A factor analysis approach was used to understand the structure of the attributes (variables) (Field 2013). This approach allows for the reduction of the number of attributes (19) to a smaller number of underlying factors (latent variables) that increase the ease of comparison and interpretation.
The factor analysis was based on a correlation matrix and was conducted with SPSS, version 22, for Windows, using the principle component extraction method with varimax rotation and Kaiser normalisation.
The survey results are suitable for factor analysis as indicated by a Kaiser–Meyer–Olkin (KMO) measure of sampling adequacy that produced a KMO measure of 0.889 (Field 2013). A KMO measure close to one indicates that correlation patterns are concentrated and thus a factor analysis will produce distinctive and trustworthy factors (Field 2013).
This is confirmed by the KMO measures for the individual variables which were all in excess of 0.6. Bartlett’s test is important in indicating that the underlying latent structure of the variables can be identified with factor analysis. The reliability of the questionnaire is satisfactory as measured by a Cronbach’s alpha (Field 2013) of 0.913. Internal consistency or reliability is measured by Cronbach’s alpha and indicates correlation among items or factors. When a strong correlation is present between items they are strongly correlated with one another and the Cronbach’s alpha will measure 0.90 or higher (Maree 2007).
To determine the number of significant factors the eigenvalues of the correlation matrix need to be examined. The measure of how much of the variance of the observed variables a factor explains is known as the eigenvalue. Therefore, an eigenvalue equals to or greater than one describes more variance (represents substantial variation [Field 2013]) than a single observed variable. A factor that defines the least amount of variable is rejected (Rahn 2015). Exploratory factor analysis on the 2013 data lead to the identification of four factors (eigenvalues > 1) that explained 65% of the variation (the results are shown in Table 1).
TABLE 1: Initial factor analysis – total variance explained. |
Deciding how many factors need to be retained is known as extraction (Field 2013). Eigenvalues indicate the importance of a factor, and it is rational to retain the factors with high eigenvalues (Field 2009). Plotting each eigenvalue against the associated factor on a graph is known as a scree plot (Cattell 1966 as cited in Field 2013). Typically, the graph will include factors with high eigenvalues and many factors with lower eigenvalues, creating a unique shape. Cattell (1966 as cited in Field 2013) suggests that the point where the slope of the line changes is the cut-off for factors to be retained.
The scree plot associated with Table 1 shows the relative importance of each factor (Field 2013), and it is apparent from Figure 5 that four factors should be retained.
Confirmatory factor analysis and factor rotation
Confirmatory factor analysis (CFA) tests how well the measured variables represent the number of factors; it specifies the number of factors required in the data and which measured variables are related to latent variables (Statistics Solutions 2015).
A CFA for four factors was subsequently conducted, and the results are shown in Table 2.
TABLE 2: Confirmatory factor analysis – four factors (2013). |
The factor loadings clearly indicate the four factors, but there are a number of attributes with significant cross-loadings. For example, Ease of getting to the airport has a loading of 0.554 on factor 1 and a loading of -0.636 on factor 2.
In order to get a clear factor structure (no significant cross-loadings), factor rotation can be used (Field 2013). The results of the factor rotation are shown in Table 3.
TABLE 3: Factor rotation – four factors (2013). |
The rotated factor matrix contained a clear factor structure, but Price of ticket recorded a significant cross-loading (> 0.4) on factors 2 and 4, as well as Ease of check-in that recorded significant cross-loadings on factors 1 and 3. In order to address this issue, the attributes associated with the significant cross-loadings (Price of ticket and Ease of check-in) were removed from the data set.
Factor analysis on the reduced data set (KMO measure = 0.89) resulted in the structure shown in Table 4.
TABLE 4: Factor rotation – four factors (2013). |
The analysis resulted in a clear factor structure, but Ease of parking did not record significant loadings (> 0.5) on any of the factors. The removal of Ease of parking from further analysis resulted in a factor structure similar to that depicted in Table 3.
The following four latent factors, depicted in Table 5, can be identified from the factor analysis results and can be grouped as follows:
- Airline efficiency and facilities (factor 1).
- Brand, safety and security (factor 2).
- Access to the airport (factor 3).
- Cost (factor 4).
TABLE 5: Latent factor structure of attributes (2010). |
Comparison of latent factors: 2010 and 2013
Heyns and Carstens (2011) completed a similar survey at Lanseria in 2010. However, the questionnaire used in their survey was based on 18 attributes such as Short check-in and Airline brand, which were included in the 2013 questionnaire, but were not included in the 2010 survey.
The latent factors obtained from the 2010 survey were similar to the 2013 latent factors as shown in Table 6.
TABLE 6: Latent factor structure of attributes (2013). |
Heyns and Carstens (2011) used factor loadings in excess of 0.7 to identify the significant attributes, whereas the attributes identified in Table 6 relate to attributes with a factor loading in excess of 0.5.
Although the factor loadings were different, the objective of factor analysis is to identify groups of variables that correlate significantly. If factor loadings in excess of 0.7 were also used for the 2013 data, the result would be as shown in Table 7.
TABLE 7: Latent factor structure of attributes. |
Various approaches may be used to compare the latent factors obtained from the two data sets (2013 and 2010). However, for comparative purposes, it is necessary to ensure that the data sets include the same attributes and to this end Short check-in was excluded from the 2013 data. An exploratory factor analysis was completed on both sets of data, and the results are shown in Tables 8 and 9.
TABLE 8: Factor structure (rotated) – 2010 data. |
TABLE 9: Factor structure (rotated) – 2013 data. |
One approach to compare the factors of the different data sets is to calculate the correlations between the factors of the two data sets. The factor correlation matrix is shown in Table 10.
TABLE 10: Factor correlation matrix (2010 and 2013). |
The information in Table 10 indicates high levels of correlation between the following factors of the two data sets:
- Factor 1 2010 and Factor 1 2013 (airline efficiency, facilities) 0.94.
- Factor 2 2010 and Factor 3 2013 (access to airport) 0.87.
- Factor 3 2010 and Factor 2 2013 (brand, safety and security) 0.97.
- Factor 4 2010 and Factor 4 2013 (cost) 0.95.
Comparing the latent factors from the 2010 data set with that of 2013 indicated similarity between the factors. Another approach to compare the factors of the two data sets and to confirm the findings of the first comparison is known as Tucker’s congruence coefficient (Lorenzo-Seva & Ten Berge 2006). The congruence coefficient can be interpreted as a measure of proportionality and is calculated as follows:

where xi and yi are the loadings of the attribute i of factors x and y, respectively (i = 1, …, n) (Lorenzo-Seva & Ten Berge 2006). The calculated congruence coefficients are shown below:
- Factor 1 2010 and Factor 1 2013 (airline efficiency, facilities) 0.98.
- Factor 2 2010 and Factor 3 2013 (access to airport) 0.93.
- Factor 3 2010 and Factor 2 2013 (brand, safety and security) 0.99.
- Factor 4 2010 and Factor 4 2013 (cost) 0.97.
Lorenzo-Seva and Ten Berge (2006) suggested that congruence coefficients ranging between 0.85 and 0.94 can be considered as factors with ‘fair similarity’, whereas congruence coefficients with a value of 0.95 and higher can be considered as identical. Therefore, the factors are considered to be equal.
From the above, it is evident that airline efficiency and facilities, brand, safety and security as well as cost are considered identical (congruence coefficient of 0.95 and higher) for both studies, while access to the airport has a reasonable similarity (congruence coefficient of 0.93).
Based on both approaches, it can be concluded that there were no differences in the way that passengers evaluated the attributes in 2010 compared to 2013. It is evident that the respondents in both surveys considered the same attributes to be influential in their choice of airport.
Thus, airline efficiency and facilities, access to airport, safety and security as well as cost are the most important attributes passengers will consider when deciding to fly from Lanseria Airport. Even after the entry of an additional LCC, the attributes respondents regarded as important, and the way in which they evaluated the attributes, in their airport choice decision remained unchanged.
Conclusion
In this research, the findings of a survey conducted at Lanseria International Airport in 2013 were discussed. These findings were compared to the findings of a similar survey conducted there in 2010.
The objective of this study was to determine the reasons passengers prefer to fly from Lanseria Airport and to determine whether these factors would differ after the entry of an additional LCC at the airport.
The survey conducted in 2013 found that respondents who resided in Gauteng – and more specifically the northern, western and central parts of the province – chose to use Lanseria Airport because it is in close proximity to them. When a comparison was drawn with the 2010 study, it was established that the respondents residing in the northern, western and central regions of Gauteng preferred to use Lanseria Airport because of the fact that it is conveniently situated near to them.
In both surveys, it was established that most of the respondents resided in Gauteng. It became evident that when airports in a metropolitan area are close to one another, passengers consider access time when selecting an airport. This finding was confirmed by plotting the residential (suburb) postal codes on a map of Gauteng.
The above-mentioned latent factors identified in the factor analysis were then compared to the latent factors resulting from the 2010 factor analysis. It was established that the factor analysis conducted by Heyns and Carstens (2011) on the 2010 data resulted in identifying the same latent factors in 2013. Based on the comparison of the 2010 and 2013 factor analysis, it was determined that even after the entry of a second LCC, respondents still considered the same attributes to be important in their airport choice decision.
Acknowledgements
Competing interests
The authors declare that they have no financial or personal relationships that may have inappropriately influenced them in writing this article.
Authors’ contributions
J.W. was the project leader and E.K. was responsible for experimental and conceptual contributions.
References
Airports Company South Africa (ACSA), 2015, Integrated annual report 2015, viewed 10 August 2016, from http://www.acsa.co.za
Barbot, C., 2006, ‘Low-cost airlines, secondary airports, and state aid: An economic assessment of the Ryanair-Charleroi Airport agreement’, Journal of Air Transport Management 12, 197–203. http://dx.doi.org/10.1016/j.jairtraman.2006.04.001
Barbot, C., 2009, ‘Airport and airlines competition: Incentives for vertical collusion’, Transportation Research 43, 952–965. http://dx.doi.org/10.1016/j.trb.2009.04.001
Barrett, S.D., 2004, ‘The sustainability of the Ryanair model’, International Journal of Transport Management 2, 89–98. http://dx.doi.org/10.1016/j.ijtm.2004.12.001
Bennet, R.D. & Craun, J.M., 1993, The Southwest effect, viewed 26 April 2012, from https://docs.google.com
Boksberger, P. & Schuckert, M., 2011, Innovationen in tourismus und freizeit: Hypes, trends und entwicklungen, viewed 05 August 2015, from https://books.google.co.za
De Neufville, R., 2005, Multi-airport systems in the era of no-frills airlines, viewed 24 February 2012, from http://esd.mit.edu
De Wit, J.G. & Zuidberg, J., 2012, ‘The growth limits of the low cost carrier model’, Journal of Air Transport Management 21, 17–23. http://dx.doi.org/10.1016/j.jairtraman.2011.12.013
European Low Fares Airline Association (ELFAA), 2004, Liberalisation of European air transport: The benefits of low fares airlines to consumers, airports, regions and the environment, viewed 29 October 2013, from http://www.elfaa.com
Field, A., 2009, Discovering statistics using SPSS, 3rd edn., Sage, London.
Field, A., 2013, Discovering statistics using IBM SPSS statistics, 4th edn., Sage, London.
FlySafair, 2015, FlySafair routes, viewed 06 August 2015, from http://www.flysafair.co.za
Goedegebuure, L., 2010, Airport selection in regions with one primary and recently emerged secondary airport, Amsterdam.
Harvey, G., 1987, ‘Airport choice in a multiple airport region’, Transportation Research Record Part A: Policy and Practice 21(6), 439–449. http://dx.doi.org/10.1016/0191-2607(87)90033-1
Hess, S., 2010, ‘Evidence of passenger preference for specific types of airports’, Journal of Air Transport Management 16, 191–195. http://dx.doi.org/10.1016/j.jairtraman.2009.11.006
Heyns, G. & Carstens, S., 2011, ‘Passenger choice decisions at a regional airport in South Africa’, Journal of Transport and Supply Chain Management 5(1), 186–201.
Innes, J.D. & Doucet, D.H., 1990, Effects of access distance and level of service on airport choice, viewed 10 October 2013, from http://www.ascelibrary.org
Institute of Transport and Logistics Studies (ITLS) (Africa), 2010, Lanseria Airport survey – Preliminary report, University of Johannesburg, Johannesburg, South Africa.
IOL, 2016, FlySafair takes off from Lanseria, viewed 20 July 2016, from http://www.iol.o.za
Jiangtao, L., 2009, Modelling passenger choice behaviour in Chinese multi-airport regions, viewed 28 October 2013, from http://ascelibrary.org
Kulula, 2013, Flight schedule, viewed 28 January 2013, from http://www.kulula.com
Leitch, G., 2011, ‘Lanseria leaps forward’, SA Flyer Lanseria Supplement edition 188.
Lorenzo-Seva, U. & Ten Berge, J.M.F., 2006, ‘Tucker’s congruence coefficient as a meaningful index of factor similarity’, Methodology 2(2), 57–64. http://dx.doi.org/10.1027/1614-2241.2.2.57
Luke, R. & Walters, J., 2013, ‘Overview of the developments in the domestic airline industry in South Africa since market deregulation’, Journal of Transport and Supply Chain Management 7(1), Art. #117, 1–11. http://dx.doi.org/10.4102/jtscm.v7i1.117
Mango Airlines, 2013, Flight schedule, viewed 28 January 2013, from http://flymango.com
Map Studio, 2010, South Africa map, viewed 30 October 2010, from http://mapstudio.co.za
Map Studio, 2013, South Africa map, viewed 28 January 2013, from http://mapstudio.co.za
Maree, K. (ed.), 2007, First steps in research, Van Schaik Publishers, Pretoria.
Marucci, E. & Gatta, V., 2011, ‘Regional airport choice: Consumer behaviour and policy implications’, Journal of Transport Geography 19, 70–84.
Mokgata, Z., 2012, Lanseria takes off, viewed 28 October 2013, from http://www.financialmail.co.za
News 24, 2012, Time is up for 1Time, viewed 11 April 2012, from http://www.news24.com
News 24, 2013, World’s 10 busiest flight routes, viewed 30 June 2014, from http://www.news24.com
Pels, E., Nijkamp, P. & Rietveld, P., n.d., Airport choice in a multiple airport region: An empirical analysis for the San Francisco Bay area, viewed 08 October 2013, from http://papers.tinbergen.nl
Rahn, M., 2015, Factor analysis: A short introduction, Part 1, viewed 17 August 2015, from www.theanalysisfactor.com
Skinner, R.E., Jr., 1976, ‘Airport choice: An empirical study’, Transportation Engineering Journal of ASCE102, 871–884.
Smith, E., 1998, An evaluation of the impact of air transport deregulation in South Africa, Rand Afrikaans University, Johannesburg.
Statistics Solutions, 2015, Confirmatory factor analysis, viewed 18 August 2015, from www.statisticssolutions.com
Transportation Dictionary, 2015, Secondary airport, viewed 05 August 2015, from http://www.transportation-dictionary.org
Traveller 24, 2015a, First Wonderboom direct flight to Cape Town takes off, viewed 01 September 2015, from www.traveller24.news24.com
Traveller 24, 2015b, Mango expands its Lanseria operations with new flight route, viewed 17 August 2016, from www.traveller24.news24.com
Vasigh, B., Fleming, K. & Tacker, T., 2008, Introduction to air transport economics, Ashgate, Hampshire, England.
Warnock-Smith, D. & Potter, A., 2005, ‘An exploratory study into airport choice factors for European low-cost airlines’, Journal of Air Transport Management 11, 388–3892. http://dx.doi.org/10.1016/j.jairtraman.2005.05.003
Wensveen, J.G., 2011, Air transportation a management perspective, Ashgate, Surrey, England.
Windle, R. & Dresner, M., 1995, Airport choice in multi-airport regions, viewed 08 October 2013, from http://ascelibrary.org
Zhang, Y. & Xie, Y., 2005, ‘Small community airport choice behaviour analysis: A case study of GTR’, Journal of Air Transport Management 11, 442–447. http://dx.doi.org/10.1016/j.jairtraman.2005.07.008
Footnotes
1. An airport that receives regular traffic (serves a town or community) as an alternative to the primary airport (Transportation Dictionary 2015) and not utilised by traditional full-service carriers (Boksberger & Schuckert 2011).
2. SA Airlink began a scheduled service from Wonderboom Airport in Pretoria to Cape Town in August 2015 (Traveller24 2015a) but was not included in this study as the fieldwork was conducted in 2013. It is also not considered to be a LCC. It should also be noted that no other city served by LCCs in SA have alternative airports to their main airports.
3. Statistical consultation service that assists researchers at the University of Johannesburg.
|